#13
Lyduine Collij
From AMYPAD to EuroPAD – creating a multi-cohort preclinical database to model AD disease trajectorie
Background: The emergence of disease-modifying drug therapies is expected to revolutionize the field of Alzheimer’s disease (AD). Recent results from anti-amyloid clinical trials highlight the importance of early identification and accurate risk-stratification of individuals in early stages of the disease. In this context, the Amyloid Imaging to Prevent Alzheimer’s Disease (AMYPAD) Prognostic and Natural History Study (PNHS) was established, leveraging existing cohorts to alleviate the burden of recruiting de novo participants1, 2. Here, we describe the harmonization and integration efforts of imaging, clinical, cognitive, and fluid biomarker data.
Access to the data is available through the Alzheimer’s Disease Data Initiative, with additional details provided at https://amypad.eu/data/
Methods: The AMYPAD-PNHS integrates prospective and historical data from 32 European sites across 10 countries currently3. These sites contribute data from 10 Parent Cohorts (PC), predominantly comprising non-demented at-risk subjects, including EPAD LCS, EMIF-AD (60++ and 90+), ALFA+, FACEHBI, FPACK, UCL-2010-412, Microbiota, DELCODE, and the AMYPAD Diagnostic and Patient Management Study (DPMS).
A meticulous data curation process was implemented, harmonizing metrics and questionnaires through strategies such as recoding into categories, Percentage of Maximum Possible Scores, and z-scores. Expert reviewers at each site conducted PET visual reads. Centralized quantification of static PET images, employing site-specific Gaussian smoothing, yielded harmonized Centiloid values. Parametric modelling of dynamic PET scans was also performed providing metrics such as the distribution volume ratio (DVR).
Results: The initial data set includes 3366 participants (55% females, 67±8 years), with 2629 having at least one follow-up visit (2.56±1.91 years). Of those, 1618 underwent baseline amyloid-PET, 888 with follow-up. The dataset incorporates clinical outcomes, biomarkers, risk factors, and other pertinent variables (Figure-1). Distribution of participants based on amyloid PET status at baseline is of 60% negative (<12CL), 24% grey-zone (12-50CL), and 16% positive (>50CL).
Conclusions: The AMYPAD-PNHS represents the largest European longitudinal dataset phenotyping individuals at risk of AD-related progression. The consortium is currently evolving into its new phase, namely the EuroPAD collaborative framework, and the dataset will be expanded in terms of variables (e.g., currently integrating GWAS and advanced MRI data) and additional cohorts.
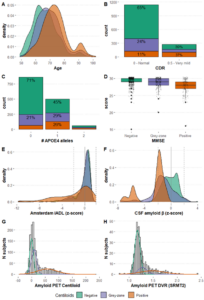
Figure 1. Representative set of variables contained harmonized and integrated into the AMYPAD PNHS dataset.
Keywords: Alzheimer’s disease, Amyloid-PET, Magnetic Resonance Imaging, consortium, disease modelling
Authors affiliations: Lyduine E. Collij, PhD1,2, David Vállez García, PhD1, Willemijn J. Jansen, PhD3, Stephanie J. B. Vos, PhD3, Julie Elisabeth Oomens, MSc4, Natalia Vilor-Tejedor, PhD5, Laura Stankeviciute, PhD5, Marta Mila-Aloma, PhD5, Anna E Leeuwis, PhD1, Gonzalo Sánchez-Benavides, PhD5, Mahnaz Shekari, MSc6, Fiona Heeman, PhD7, Luigi Lorenzini, MSc1, Leonard Pieperhoff, MSc1, Bert Overduin, PhD8, Isadora Lopes Alves, PhD9, Juan Domingo Gispert, PhD5, Frank Jessen, MD10, Pieter Jelle Visser, MD, PhD1, Anouk den Braber, PhD1, Craig W Ritchie, MBChB, PhD11, Mercè Boada, MD, PhD12, Marta Marquié, MD, PhD13, Rik Vandenberghe, MD, PhD14, Emma S. Luckett, PhD15, Michael Schöll, PhD7, Giovanni B. Frisoni, PhD, MD16, Bernard J Hanseeuw, MD, PhD17, Lisa Quenon, PhD17, Andrew W. Stephens, MD, PhD18, Lisa Ford, MD19, Mark E Schmidt, MD20, Cindy Birck, PhD21, Anja Mett, BSc22, Rossella Gismondi, MD18, Richard Manber, PhD23, Sylke Grootoonk, PhD23, Robin Wolz, PhD24, Gill Farrar, PhD25, Frederik Barkhof, Prof1,26 on behalf of the AMYPAD Consortium
(1)Amsterdam UMC, Amsterdam, Netherlands, (2)Lund University, Lund, Sweden, (3)Maastricht University, Maastricht, Netherlands, (4)Alzheimer Center Limburg, School for Mental Health and Neuroscience, Maastricht University, Maastricht, Netherlands, (5)Barcelonaβeta Brain Research Center (BBRC), Barcelona, Spain, (6)Barcelonaβeta Brain Research Center (BBRC), Pasqual Maragall Foundation, Barcelona, Spain, (7)University of Gothenburg, Gothenburg, Sweden, (8)Aridhia Informatics Ltd, Glasgow, United Kingdom, (9)Brain Research Center, Amsterdam, Netherlands, (10)German Center for Neurodegenerative Diseases (DZNE), Bonn, Germany, (11)Scottish Brain Sciences, Edinburgh, United Kingdom, (12)Ace Alzheimer Center, Barcelona, Spain, (13)Ace Alzheimer Center Barcelona, Barcelona, Spain, (14)University Hospitals Leuven, Leuven, Belgium, (15)Karolinska Institutet, Stockholm, Sweden, (16)University of Geneva, Geneva, Switzerland, (17)UC Louvain, Brussels, Belgium, (18)Life Molecular Imaging GmbH, Berlin, Germany, (19)Janssen Pharmaceutica, Titusville, NJ, USA, (20)Janssen Pharmaceutica, Beerse, Belgium, (21)Alzheimer Europe, Luxembourg, Luxembourg, (22)GE Healthcare, Amersham, United Kingdom, (23)IXICO, London, United Kingdom, (24)IXICO, London, Greater London, United Kingdom, (25)GE HealthCare, Amersham, United Kingdom, (26)University College London, London, United Kingdom
References:
- Lopes Alves I, Collij LE, Altomare D, et al. Quantitative amyloid PET in Alzheimer’s disease: the AMYPAD prognostic and natural history study. Alzheimers Dement 2020;16:750-758.
- Collij LE, Farrar G, Vallez Garcia D, et al. The amyloid imaging for the prevention of Alzheimer’s disease consortium: A European collaboration with global impact. Front Neurol 2022;13:1063598.
- Bader I, Bader I, Lopes Alves I, et al. Recruitment of pre-dementia participants: main enrollment barriers in a longitudinal amyloid-PET study. Alzheimer’s Research & Therapy 2023;15:189.